Once you listen to the music, you can know the score, you can play it right away, and you can also master the “eighteen instruments”, such as piano, violin, and guitar. This is not a human music master, but a “multi-task and multi-track” music-to-note model MT3 launched by Google.
First, need to explain what multitasking and multitracking are. Usually, a piece of music comes from an ensemble of multiple instruments, each piece is a track, and multitasking is to restore the scores of different tracks at the same time.
In fact, Google MT3 has achieved SOTA results in restoring multi-track music scores. Google has submitted the paper to ICLR 2022.
JOIN US ON TELEGRAM
Restore multi-track music score
Compared with automatic speech recognition (ASR), automatic music transcription (AMT) is much more difficult, because the latter not only needs to transcribe multiple instruments at the same time but also retains fine pitch and time information.
The multi-track automatic music transcription data set is even more “low-resource”. Existing open-source music transcription data sets generally only contain one to a few hundred hours of audio, which is very small compared to the market where voice data sets are often tens of thousands of hours.
The previous music transcription mainly focused on task-specific architecture, tailored to the various instruments of each task. Therefore, the author was inspired by low-resource NLP task transfer learning and proved that the general Transformer model can perform multi-task AMT and significantly improve the performance of low-resource instruments. The author uses a single general Transformer architecture T5 and is a T5 “small” model, which contains approximately 60 million parameters.
This model uses a series of standard Transformer self-attention “blocks” in the encoder and decoder. In order to generate the output tag sequence, the model uses greedy autoregressive decoding: input an input sequence, append the output tag with the highest predicted probability to the sequence and repeat the process until the end.
Moreover, MT3 uses Mel spectrogram as input. For output, the author constructed a token vocabulary inspired by the MIDI specification, called “MIDI-like”.
The generated score is rendered into audio by the open-source software FluidSynth. In addition, it is necessary to solve the problem of the unbalanced and different structures of different music data sets.
The universal output token defined by the author also allows the model to be trained on a mixture of multiple data sets at the same time, similar to the simultaneous training of several languages with a multilingual translation model. This method not only simplifies model design and training but also increases the amount and variety of training data available for the model.
Actual effect
On all indicators and all data sets, MT3 consistently outperforms the baseline. The data set mixture during training has a great performance improvement compared with the training of a single data set, especially for “low resource” data sets such as GuitarSet, MusicNet, and URMP.
Recently, the Google team also released the source code of MT3 and released a demo on Hugging Face. However, since the conversion of audio requires GPU resources, on Hugging Face, it is recommended that you run Jupyter Notebook on Colab.
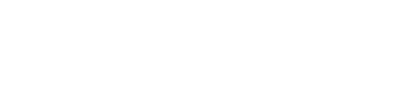